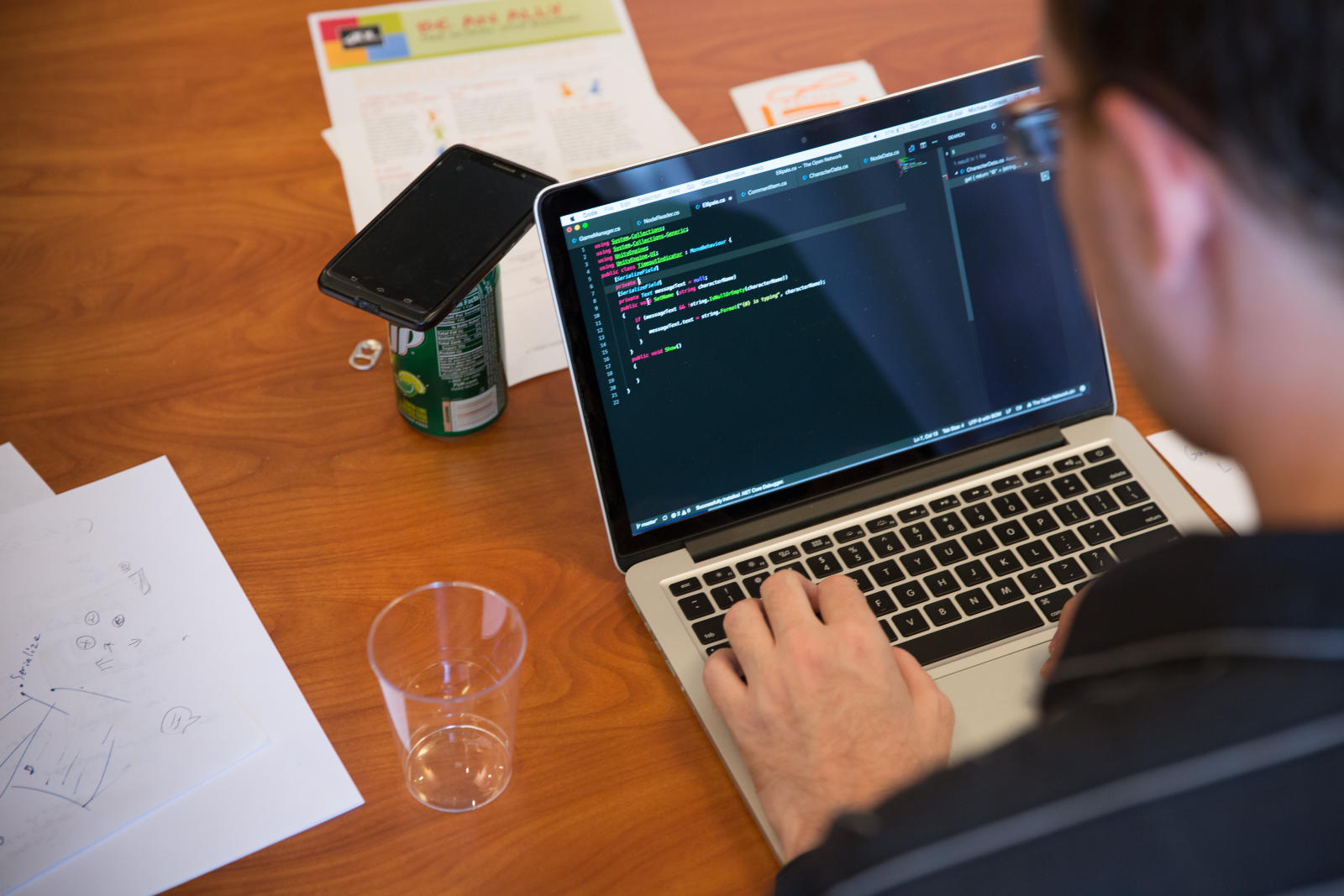
ADL hosted its first Game Jam in October 2017, where game creators in 7 countries, across 4 continents, and from live events in 3 U.S. locations created video games based on substantive guidance from ADL staff about allyship. October 2017, CSC office
New York/Berkeley, Calif. February 6, 2018…
The Anti-Defamation League’s (ADL) Center for Technology and Society today announced preliminary results from an innovative project that uses artificial intelligence, machine learning, and social science to study what is and what isn’t hate speech online. The project’s goal is to help the tech industry better understand the growing amount of hate online.
The Center for Technology and Society (CTS) has collaborated with the University of California at Berkeley’s D-Lab since April 2017 to develop the Online Hate Index. ADL and the D-Lab have created an algorithm that has begun to learn the difference between hate speech and non-hate speech. The project has completed its first phase and its early findings are described in a report released today. In a very promising finding, ADL and the D-Lab found the learning model identified hate speech reliably between 78 percent and 85 percent of the time.
“For more than 100 years, ADL has been at the forefront of tracking and combating hate in the real world. Now we are applying our expertise to track and tackle bias and bigotry online,” said ADL CEO and National Director Jonathan Greenblatt. “As the threat of cyberhate continues to escalate, ADL’s Center for Technology and Society in Silicon Valley is convening problem solvers and developing solutions to build a more respectful and inclusive internet. The Online Hate Index is only the first of many such projects that we will undertake. U.C. Berkeley has been a terrific partner and we are grateful to Reddit for their data and for demonstrating real leadership in combating intolerance on their platform.”
“This project has tremendous potential to increase our ability to understand the scope and spread of online hate speech,” said Brittan Heller, CTS’s director. “Online communities have been described as our modern public square. In reality though, not everyone has equal access to this public square, and not everyone has the privilege to speak without fear. Hateful and abusive online speech shuts down and excludes the voices of the marginalized and underrepresented from public discourse. The Online Hate Index aims to help us understand and alleviate this, and to ensure that online communities become safer and more inclusive.”
The research led to several other interesting findings, including the fact that when searching for one kind of hate, it’s easy to find hate of all kinds. In the initial results, there were several words that appeared more frequently in hate speech than non-hate speech. The top five words most strongly associated with hate were: Jew, white, hate, women, and black.
The project also found patterns in the construction of hateful language.
- The average number of words in a hateful comment was typically longer than in non-hateful comments.
- There were slightly more words in all caps found in hateful comments than in non-hateful ones.
- The sentence length in hateful comments was slightly longer than in non-hateful comments.
The goal of the Online Hate Index is to examine speech from multiple social media sites and develop a model that will help companies better understand the extent of hateful content on their platforms by creating community-based definitions of hate speech.
For the first phase of the project, researchers collected 9,000 comments from a handful of communities on Reddit during two months in 2016. They chose to start their research with Reddit because of the site’s community structure, its large volume of easily accessible comments, and because speech on the platform is typical of what is seen in everyday conversations, both online and offline. In future phases of the study, the researchers intend to apply their findings to speech on other social media platforms.
At the same time, the D-Lab developed a social science methodology based on a specific definition of hate speech. The lab then assembled a team of researchers with diverse backgrounds, trained them on the definition and methodology, and then manually labeled each of the comments as either hate or not hate.
Once the researchers completed labeling the comments, they fed them into the machine learning model. The model established rules after evaluating a number of examples of what people have classified as hate speech or not hate speech.
“The machine learning algorithms can decipher whether text is hate speech or not.” said Claudia von Vacano, Executive Director of the D-Lab and the Digital Humanities at U.C. Berkeley. “Therefore, the Online Hate Index model does not have a static definition, but instead ingests labelled data that informs the predictive model.”
The next phase of the project will go beyond this simple hate analysis and evaluate specific populations in a more detailed manner. Additionally, the D-Lab will identify strategies to scale the process for labeling comments to deploy the model broadly. While there is still a long way to go with AI and machine-learning-based solutions, ADL and the D-Lab believe the technologies hold promise that we may find new ways to curb the vast amount of online hate speech.
Read more about the Center for Technology and Society at www.adl.org/CTS.